
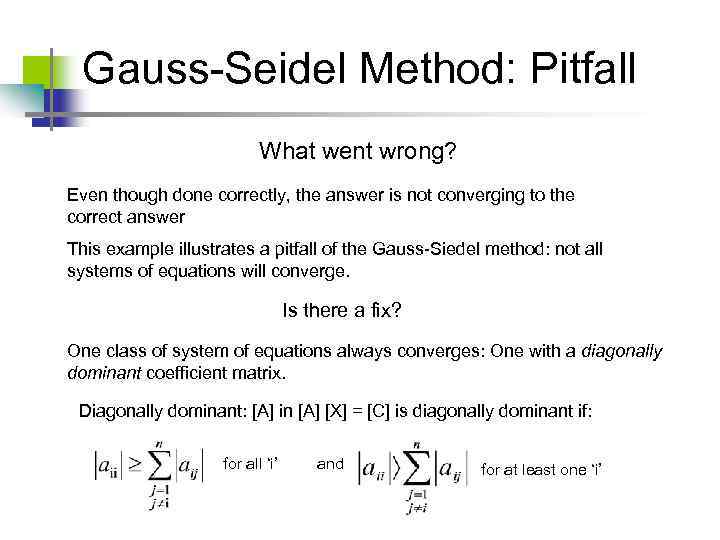
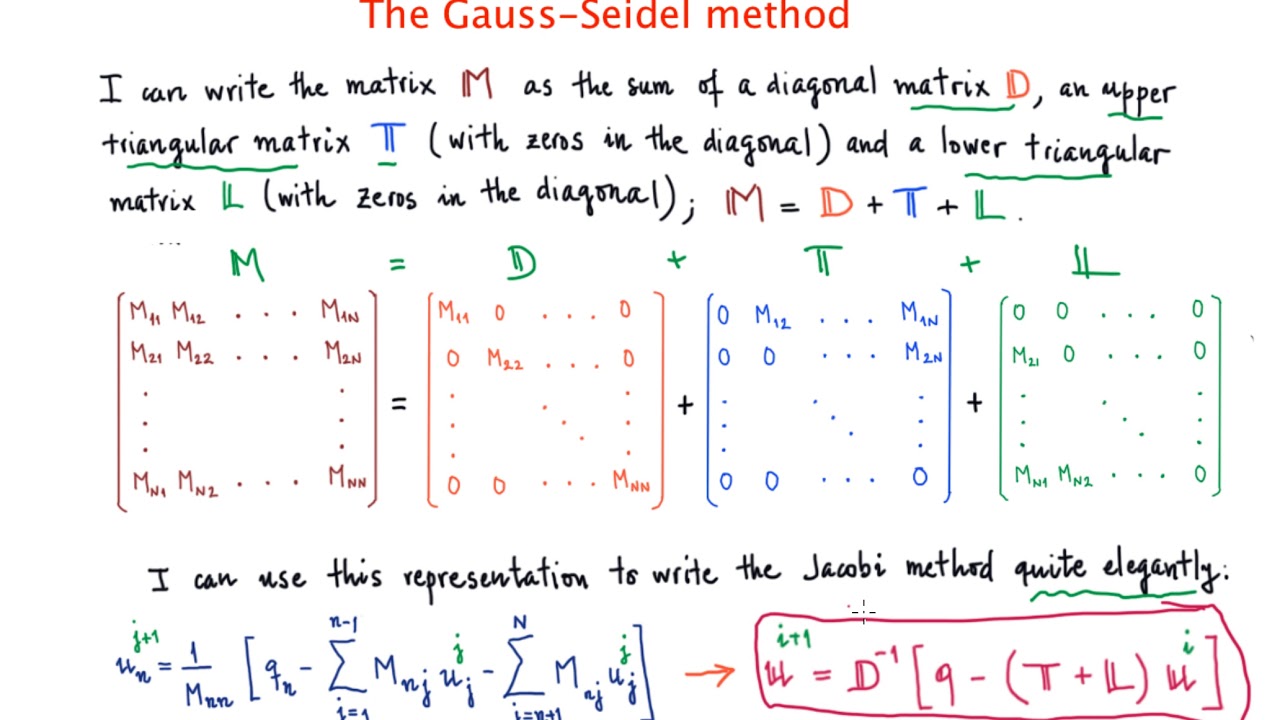
The speed drawbacks in Numpy are more pronounced for the full version (17s vs. The full algorithm uses successive overrelaxation and a checkerboard iteration scheme to improve speed and remove solver directionality, but for purposes of simplicity I provided this easier to understand version. This is actually not the full algorithm which I use. It finishes when the maximum difference between updates on the mesh is less than the indicated tolerance. The algorithm simply solves the discretized Laplace equation on a rectangular mesh (in cylindrical coordinates). I would like to use Numpy, however, since this code is part of a larger program, the almost twice as long simulation time is a significant drawback. The Matlab code runs in ~20 s whereas the Numpy codes takes ~30 s.
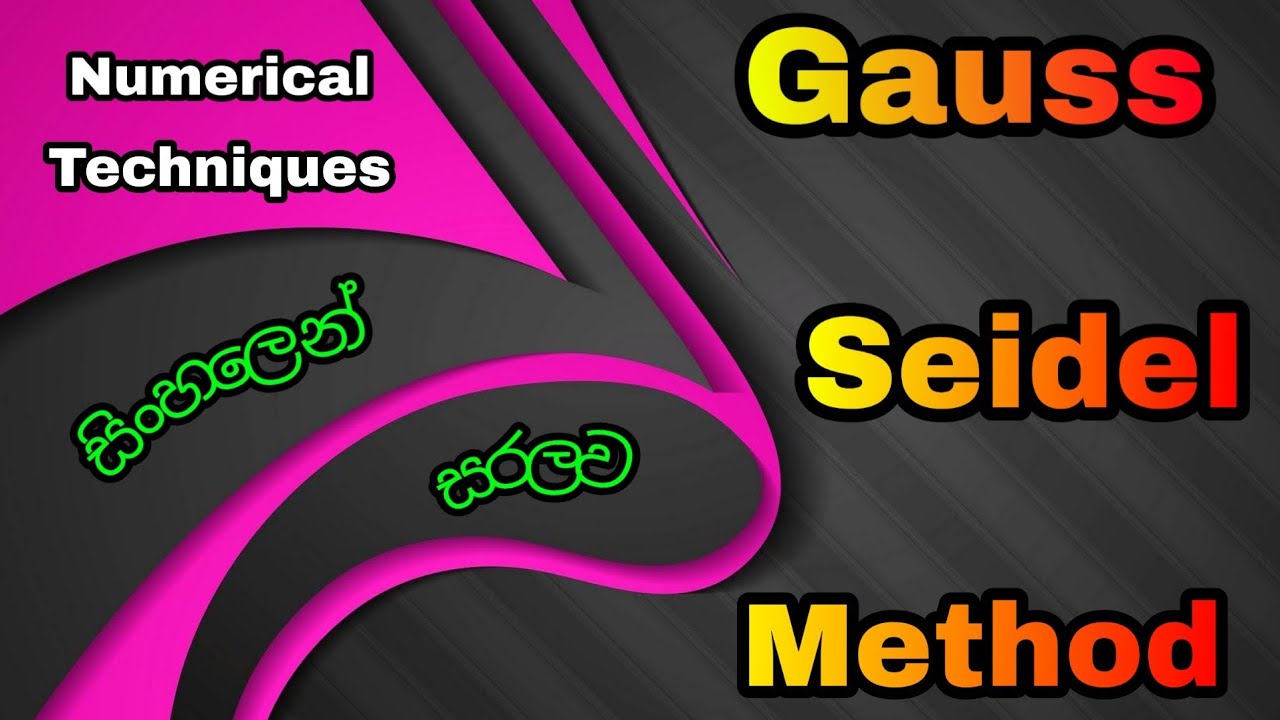
The code was originally written in MATLAB and then transferred to Python. I currently have a Gauss-Seidel solver implemented in both MATLAB and Numpy which acts on a 2D axisymmetric domain (cylindrical coordinates). XIni = input("Enter initial guesses (Must be a column vector: \n") Įrror("Partial Pivoting does not make the coefficient matrix a diagonally dominant matrix.")ĭisp("Partial Pivoting makes the coefficient matrix a diagonally dominant matrix.This question is a follow-up to a recent question posted regarding MATLAB being twice as fast as Numpy. ShowSteps = false %true shows calculation stepsĪ = input( 'Please Enter the Co-efficient Matrix, A:\n' ) ī = input( 'Please Enter the Constants(Must be a column vector, b:\n')
